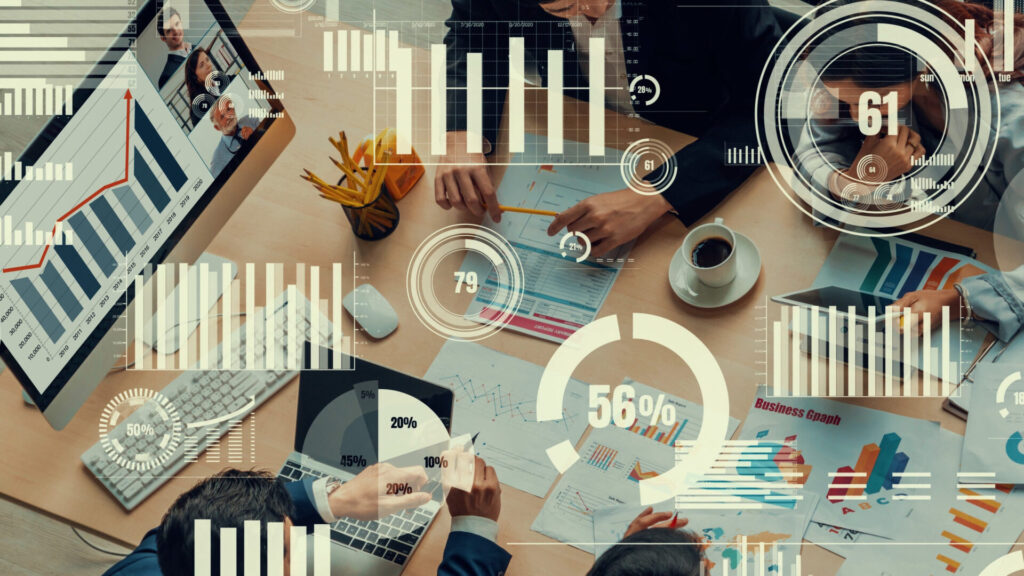
Data governance is a crucial element for modern organizations. It ensures data is used effectively, securely, and responsibly. Within the scope of data governance, implementing a strong data quality framework is particularly vital. High-quality data supports informed decision-making, regulatory compliance, and business growth. Conversely, poor data quality can lead to inefficiencies, costly mistakes, and reputational damage.
To build a strong data governance strategy, organizations must prioritize a comprehensive data quality framework. This involves using tools, setting standards, and defining processes. This article will explore how a data quality framework contributes to a successful data governance strategy.
This article might be of interest: 5 Key Factors to Consider When Establishing a Data Governance Strategy.
Why a Data Quality Framework Matters in Data Governance
A data quality framework refers to the structured approach used to ensure data is accurate, complete, consistent, and reliable. Poor data quality can negatively impact operational efficiency and strategic decisions. For example, Gartner found that organizations lose an average of $12.9 million annually due to poor data quality (source: Gartner).
A strong data quality framework enables organizations to trust the insights derived from their data. Trustworthy data leads to more reliable analytics and reporting. It also strengthens compliance with regulatory requirements such as GDPR and HIPAA. To achieve these benefits, organizations must actively manage and improve their data quality within a defined framework. Here are three best practices that should be followed.
Best Practices for Building a Data Quality Framework
1. Use Data Quality Monitoring Tools and Conduct Regular Audits
Monitoring tools are essential for identifying and addressing data quality issues in real time. These tools provide automated alerts for anomalies, duplicate entries, or missing data.
Regular audits complement monitoring tools by providing a more in-depth review of data quality. Audits can uncover systemic issues that automated tools might miss. For example, a financial services company might audit its customer database quarterly. During the audit, they identify outdated contact information and correct it before launching the next new campaign.
Conducting regular audits ensures that data remains consistent and up-to-date. It also helps organizations spot trends in data quality issues and address their root causes. Both monitoring tools and audits are critical components of a robust data quality framework.
2. Define Standards That All Departments Follow to Maintain Data Integrity
A lack of standardized practices across departments often results in inconsistent data. To avoid this, organizations should establish clear data quality standards within their framework. These standards need to define how data is collected, stored, processed, and shared.
For example, a retail company might standardize how customer information is entered into its system. All departments must follow specific rules for formatting, such as consistent phone number formats and complete address entries. This minimizes errors and ensures data consistency. This is an example of GIGO, or “Garbage In, Garbage Out,” which applies to data quality.
Documentation of these standards is critical. Organizations should maintain a data governance manual or repository that outlines their practices. Training employees on these standards further ensures compliance across teams. By adopting a uniform approach, businesses can maintain data integrity and prevent discrepancies. A data quality framework thrives on clearly defined and enforced standards.
3. Set Processes to Quickly Identify and Correct Data Issues Through Reporting
Even with monitoring tools and standards in place, data issues can arise. When they do, it’s essential to have well-defined reporting processes within the data quality framework. These processes should enable employees to report issues promptly and ensure swift resolution.
For example, a healthcare organization might implement a data issue reporting portal. Staff can log discrepancies, such as duplicate patient records, directly in the portal. A dedicated team then investigates and resolves these issues within a set timeframe. This approach minimizes disruptions and maintains the reliability of critical data systems. It also provides a standard response of what to do when a data quality issue arises.
Effective reporting processes require clear roles and responsibilities. Data stewards or data quality teams should oversee the resolution of reported issues. Organizations should also establish service-level agreements (SLAs) to ensure timely resolution. For instance, an SLA might mandate resolving high-priority issues within 24 hours. Such processes form an essential part of any data quality framework.
Combining Tools, Standards, and Processes for Maximum Impact
While each of these practices is valuable individually, they are most effective when combined as part of a data governance strategy. Monitoring tools detect problems in real time, while audits uncover deeper issues. Standards provide a framework for consistent data management across departments. Reporting processes address issues promptly and maintain operational efficiency.
Together, these practices form a robust data quality framework. Organizations that adopt these strategies can build a foundation of trustworthy data. This foundation supports better decision-making, stronger compliance, and long-term business success.
Real-World Examples of an Effective Data Quality Strategy
Organizations across industries have successfully implemented data quality frameworks. For instance, Coca-Cola’s data governance strategy focuses on standardization and quality monitoring. The company uses tools to ensure its data remains consistent across global operations (source: Forbes).
Healthcare organizations, such as Kaiser Permanente, now employ rigorous data quality audits and reporting processes. These efforts ensure patient data remains accurate and secure. As a result, the organization has improved care outcomes and operational efficiency.
It should now be clear the importance of combining technology, standards, and processes as part of an overall data governance strategy. By doing so, organizations can overcome data quality challenges and achieve their governance goals through an effective data quality framework.
Building and maintaining quality data is essential for a successful data governance strategy. Organizations must use monitoring tools, conduct audits, define standards, and establish reporting processes within this framework. These practices ensure data remains accurate, consistent, and reliable.
A comprehensive data quality framework is a cornerstone of effective decision-making and regulatory compliance. It also drives operational efficiency and supports business growth. By prioritizing a data quality framework, organizations can build a solid foundation for long-term success.